Artificial Intelligence (AI) and Machine Learning (ML) are rapidly evolving fields, showing transformative potential across domains including aviation with successes in flight prediction tasks, advanced aircraft design and predictive maintenance. Yet they face challenges related to data quality, explainability and computational demands, with the ability to generalise on new data being key for ensuring effectiveness across various real-world scenarios.
Bauhaus Luftfahrt analysed emerging AI technologies with high potential to address these hurdles. Generative AI enables the creation of realistic data examples, enhancing data quality, while transformers boost interpretability, scalability and generalisation. Their capacity to handle large multimodal datasets, incorporate domain knowledge and accurately solve unseen tasks through semantic understanding sets performance benchmarks in a growing range of applications beyond production and maintenance.
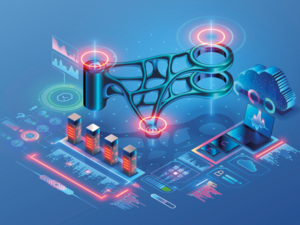
Moreover, physics-based ML integrates physics principles with data in ML models, enhancing reliability and interpretability, while reducing data needs, as internal relationships replicate physical laws. This approach excels in addressing complex multi-physics challenges such as optimising aerodynamic performance or electric motor or heat exchanger design, providing robust predictions even with incomplete knowledge of the laws of physics.
Compared to traditional mesh-based simulations, computing effort and time are significantly reduced towards real-time processing with at least similar accuracy. This technology shows high potential for generative design, enabling the efficient creation of new, optimised designs within constraints over vast design spaces, even where methods based on traditional high-fidelity simulations are infeasible.
Physics-informed neural network training for optimisation tasks
For example, within generative design this training enables the approximation of the airflow around an airfoil at any new domain point without a mesh – while simultaneously improving its parameters for an optimal lift-to-drag ratio with adaptive sampling in error-prone regions to enhance accuracy.
Application benchmarks – relative difference in error and run-time acceleration
Physics-informed ML accelerates complex physics simulations beyond traditional numerical as well as ML-based methods, achieving at least similar accuracy with significant potential for design space exploration and generative design.